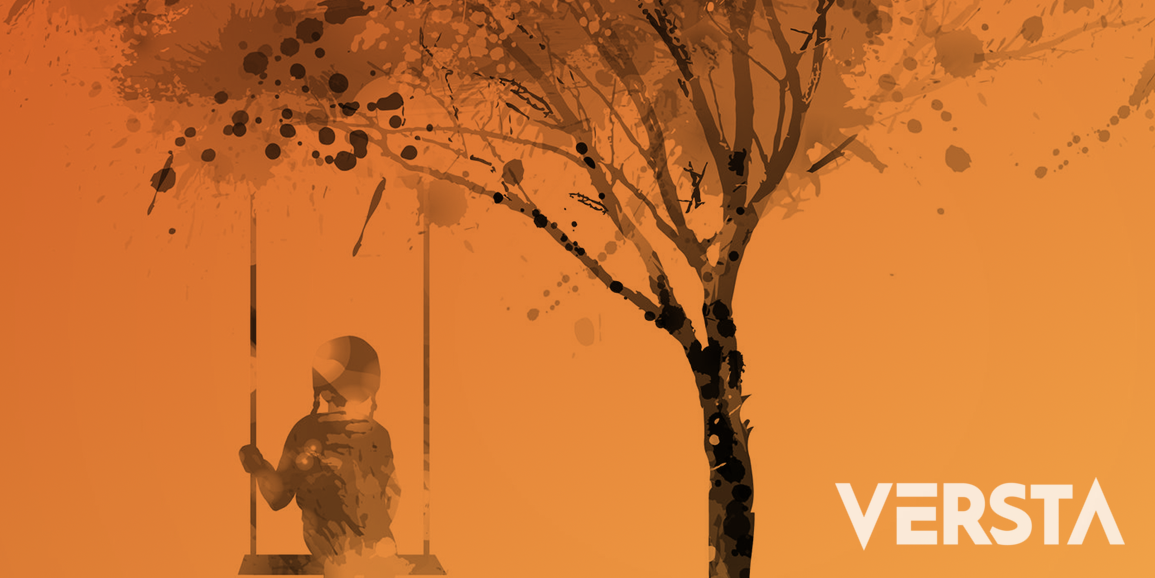
June 2011
Dear Reader,
One of the most common questions we get from both research and marketing professionals is, “What sample size is needed for the research I want to do?” The answer always depends on the type of study, the objectives of the study, the audience to whom it will be presented, and of course budget. We can make legitimate statistical calculations based on any sample size, but there are dramatic trade-offs in precision and cost no matter what sample size is chosen.
For this newsletter, we built an interactive graph for choosing sample size. It illustrates how margin of error changes as sample size changes. It will give you, your team, and your internal clients a more intuitive grasp of why very small samples can be risky and very large samples can be wasteful.
Other items of interest in this newsletter include:
- Avoiding the pitfalls of nutty Net Promoter Scores
- Online surveys have the same accuracy as phone
- Lessons from Dilbert on the perils of research
- How data can highlight mistakes
- Don’t be the ‘Me’ Generation with your surveys
- Advice for PR surveys: Avoid numeric scales
- The myth of too many choices
- Reasons to avoid grid-format questions
- How to find gold in your data mine
- What people think of surveys
- What you may need is marketing, not market research
- Overcoming your math curse
We are also delighted to share with you:
You can do research with just ten people, or with ten million people. What difference will it make? About ± 31 percentage points. Please read on.
Happy Summer,
The Versta Team
An Interactive Graph for Choosing Sample Size
Two-thirds of Americans (68%) do not believe that a sample of 2,000 people can accurately reflect the views of the nation’s population (Public Opinion Quarterly, April 2011). This is mind boggling. It is easy to prove that statistical sampling works, and there are volumes of evidence in our daily lives that it works. Still, most Americans do not believe it.
In some ways, though, it makes sense that they do not believe it. A sample of 2,000 Americans is just one one-thousandth of 1% (.00001) of the full U.S. population. How can this small number possibly predict how everyone feels? In our view, big questions about whether sampling works—and smaller questions about the right sample size for specific research—will likely never go away because even though mathematical proofs and confirming evidence are there, the math and the numbers behind these questions can be quite mind boggling.
Here’s a nice chart showing the maximum margin of error you will get for sample sizes ranging from 1 to 9,999 based on a 95% confidence interval:
There is not much to be gained by increasing the sample size beyond ten thousand, going further to the right. But wouldn’t it be nice to actually show how little we decrease the margin of error by interviewing ten thousand people, 10 million people, or all 310 million Americans?
Turns out, that is nearly impossible to show. The numbers needed for effective sampling are miniscule compared to the numbers of people they ultimately represent. If your monitor is displaying this chart 5 inches wide, the chart would have to extend more than two miles to the right to reach 310 million. It would take you a brisk 40 minute walk to read the whole chart. To show a sample size of 10 million people (a mere 3% of the U.S. population) we would have to extend the chart more than the length of a football field. We thought it would be cool and educational to show you the whole chart. But the mind boggling size of the numbers gets in the way.
What if we adjust the scale, so that all 310 million fit on the chart? The problem then is that the chart shows nothing. It is a vertical line on the left, a flat line along the bottom and not much else, because all the action where sample size makes a difference happens in a tiny slice of the chart that is invisible to the naked eye:
Faced with the impossibility of a meaningful chart displaying the margin of error for all sample sizes, we built an interactive chart that allows a viewer to click and progressively zoom in to the tiny slice of the chart where sample size really matters. The first chart above does that. Move the pointer along the curve to determine the margin of error for any specific sample size. Click and drag the pointer to highlight just a slice of the graph, and it will zoom to that slice. Keep zooming as close as you want, or double click to revert to the original.
This chart does not, however, go all the way out to 310 million. Even with a zoom-able chart, the numbers are too big (Internet browsers cannot handle 310 million data points on one interactive chart). And more importantly, it just wouldn’t matter. That’s the crux of the sampling story. Adding another ten thousand, one million, or ten million people into your sample does not matter. It does not get you much closer to an accurate estimate because you’re already there with just 2,000.
Perhaps an interactive chart like this can make statistics and sampling a bit more engaging for those who feel cursed by math. At the very least, the chart will hint at the mind boggling nature of the numbers, and help you ponder the implications of an efficient and effective sample size. Go ahead with zooming in and zooming out, noting how fast or slow the margin of error changes and the slope of the curve, and consider these key points when it comes to sampling and sample size:
- If you have the budget for a large sample, don’t even consider going beyond a couple thousand, as you gain so little. Beyond a sample size of 2,000 (which gives you a margin of error of about ±2%) you would have to pull an additional 4,700 into your sample (for a total of 6,700) to gain just one more percentage point in precision. The benefit of doing this will almost never exceed the cost of including that many more people in your sample.
The exception: If you need to understand segments or subgroups within your sample, choose your sample size based on the precision you need for those smaller groups, not the sample overall. - With a small sample there is a substantial gain in precision for every random person you add to the sample. The difference between a sample of 1,000 and 1,075 is relatively small, decreasing the maximum margin of error by just a tenth of a percentage point. But the difference between a sample of 50 and 125 is dramatic, decreasing the maximum margin of error by more than five percentage points. Another twenty five, fifty, or one hundred respondents can make an important difference when you’re at the left end of the chart.
- The most important decisions you need to make happen in the relatively tiny area between about 100 and 1,200. For each additional 50 or 100 people you include in your sample, you gain a decent improvement in the precision of your estimates. So the questions to consider are always (1) how much precision do you really need, and (2) what is the cost of including each additional person?
In academic journals you will find studies with sample sizes as small as thirty to fifty people. Some of the healthcare research we do relies on samples sizes of fifty to one hundred. Most other research we do, including that for publication in media outlets, relies on samples ranging from 300 to 1,200. As for what others consider to be appropriate sample sizes, we scanned for a handful of recently published public opinion polls:
- The latest national phone survey by the Pew Research Center for the People & the Press, was conducted May 5-8, 2011. Sample size: 1,003.
- The Gallup daily tracking telephone poll tracks presidential approval ratings, among other topics. Sample size: 1,500.
- An online healthcare poll of women by Harris Interactive was conducted between April 18-20, 2011. Sample size: 1,083.
- The most recent New York Times/CBS News national poll, based on telephone interviews, was conducted May 2 and 3, 2011. Sample size: 532.
- A recent state-wide poll in Illinois was conducted by the Chicago Tribune from August 28 through September 1, 2010. Sample size: 600.
The chart, by the way, is based on simple, open source java script that can be used to display other types of marketing and market research data. It is an especially useful way to visualize data if you have thousands of data points and want to show the big picture while being able to drill down into details. Here are more examples. Many thanks to Ben Wang for his programming, advice, and consultation.
Of course this chart, as fun as it is, will not tell you exactly the sample size to choose within that range from 100 to 1,200 because crucial external factors come into play as well. The cost-benefit ratio of a larger sample size shifts rapidly from one end of this range to the other. The tipping point will depend on the particulars of your research study, including its purpose, your audience, and how difficult it is find appropriate respondents.
But if only there were a magic number for sample size, whether it be thirty, one hundred, three hundred, or one thousand, right? Well, don’t despair. Try this magic number: (312) 348-6089. We have a great deal of experience choosing sample sizes and consulting with research, marketing, and communications teams on the key questions that need to be answered within constraints of time and budget. As always, the magic is not in any number itself, but in the highly skilled way in which it is deployed and then turned from data into stories.
Some statistical fine print. The interactive chart shows maximum margins of sampling error (commonly referred to as margin of error) for various random sample sizes at the 95% confidence level. But there are multiple sources of potential error in surveying not accounted for in the chart. Such sources include coverage error, nonresponse error, measurement error, and post-survey processing error. In most cases it is not possible to quantify these additional sources of error.
Stories from the Versta Blog
Here are several recent posts from the Versta Research Blog. Click on any headline to read more.
Avoiding the Pitfalls of Nutty Net Promoter Scores
NPS is an effective measurement tool, but there are important situations where a valid and reliable survey will give low scores despite good customer service.
Online Surveys Have Same Accuracy as Phone
A new study presented at the 2011 AAPOR meetings shows that rigorously executed surveys using opt-in Internet panels are as accurate as RDD phone surveys.
Lessons from Dilbert on the Perils of Research
This cartoon highlights important lessons about market research regarding customer satisfaction research, data mining, and ethics in research.
How Data Can Highlight Mistakes
It is crucial for senior researchers to have real access to project data, as visibility into the data will highlight important errors that need to be fixed.
Don’t Be the ‘Me’ Generation with Your Surveys
To engage your customers’ participation in satisfaction surveys, give specific examples of changes you have made in the past based on previous feedback.
Advice for PR Surveys: Avoid Numeric Scales
When designing surveys for public relations, it is best to avoid numeric response scales, as they make it difficult to present a clear and simple story.
The Myth of Too Many Choices
A recent review of choice experiments shows that the idea of choice-overload is overstated, and in some circumstances more choice is better.
Reasons to Avoid Grid-Format Questions
Recent research shows that grid-type questions in surveys decrease the reliability of survey data, even if respondents are trying to give thoughtful answers.
How to Find Gold in Your Data Mine
Technology alone cannot find hidden insights and relevant patterns in databases. The key is to approach your analysis with specific questions or dream headlines.
What People Think of Surveys
Recent data shows declining enthusiasm for participating in polls and research surveys because too many surveys are uninteresting and offer no benefit to respondents.
What You May Need Is Marketing, Not Market Research
Market research is sometimes a crutch (“we just need more data!”) that detracts from the need for aggressive marketing initiatives. Here are three ways to know.
Overcoming Your Math Curse
If you want to do great quantitative market research you need to know the math. Here are four great resources to build a solid foundation and keep on learning.
Recently Published
Research on Clergy Health Factors
In 2008 and 2009, Versta Research’s president led a four-phase comprehensive research study for the General Board of Pension and Health Benefits (GBOPHB) of the United Methodist Church. The goal was to identify systemic causes of poor health among clergy. Results from this research have been published by GBOPHB and the General Board of Higher Education and Ministry.
Versta Research in the News
MORE VERSTA NEWSLETTERS